Deep learning has been used for a wide range of applications, from facial recognition to natural language processing. Now, researchers have demonstrated that this technology can also be used to assess the interior of a material simply by analyzing its surface.
This innovative approach could revolutionize how engineers and materials scientists analyze internal structures, voids, and cracks without the need for destructive testing or costly imaging techniques.
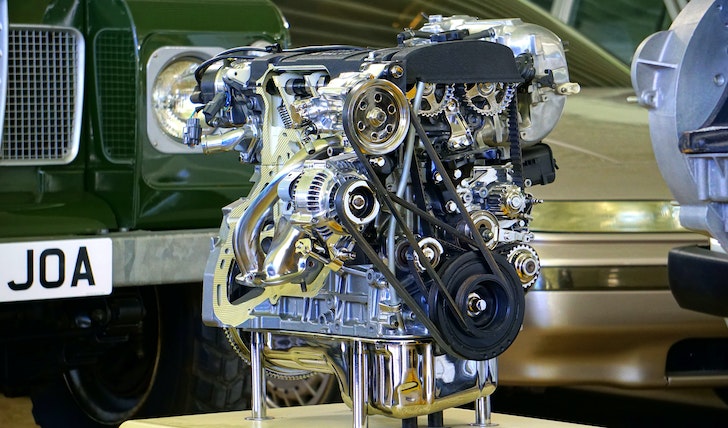
In their study, the team presented their method, which uses deep learning based on data about external force fields. By using simulations, they were able to compare different materials’ surfaces to create an accurate inference of what was going on inside them.
The researchers suggest that their deep learning model has a high accuracy rate when predicting the physical properties of a material. Say, the stiffness of the material. They also show that this approach could be used to identify appropriate materials for particular applications. And explain the underlying mechanisms at work.
Technology Is Making Engineering Easy!
Thus, the progress of deep learning has made the task of engineers unprecedentedly easy. It offers engineers the ability to assess a material’s internal structures, voids, and cracks from its surface. Based on that, engineers can now make more informed decisions with greater precision.
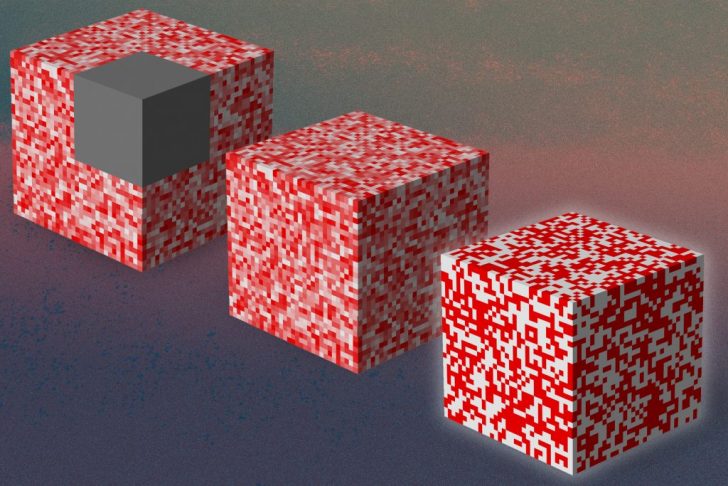
By using simulations to compare different materials’ surfaces, deep learning technology can accurately determine physical properties – for example, stiffness. Eventually, this allows engineers to select appropriate materials for specific applications.
Additionally, this approach is able to explain the underlying mechanisms of different materials and their behavior under certain conditions. Deep learning technologies also have the capability to reduce costly imaging techniques or destructive testing that was used previously in order to understand internal structures.
This new approach enables engineers and materials scientists to conduct faster assessments at a lower cost while also maintaining accuracy and efficiency.
Making Engineering Cost-Effective & Reliable
The team believes that their findings could lead to more efficient and cost-effective methods of testing materials in the future. This could potentially speed up the process of creating new products or improving existing ones.
It may even allow engineers to observe a material’s wear and tear quicker and more accurately, consequently helping them understand how it may behave over time.
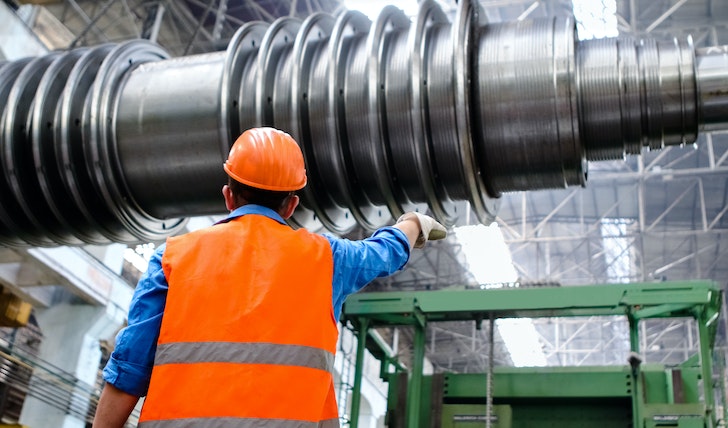
Overall, this novel deep learning method has demonstrated great potential for accurately assessing the internal features of a material from its surface data alone. With further development, it could become an invaluable tool in helping researchers better understand the inside of a material. All without costly and destructive testing.
Thus, with deep learning technology, engineers have been given an unprecedented boost in assessing materials for particular applications quickly and accurately. These advances in machine learning could revolutionize how engineering is conducted. And open up potential new areas of research.